- August 16, 2021
- Posted in AI
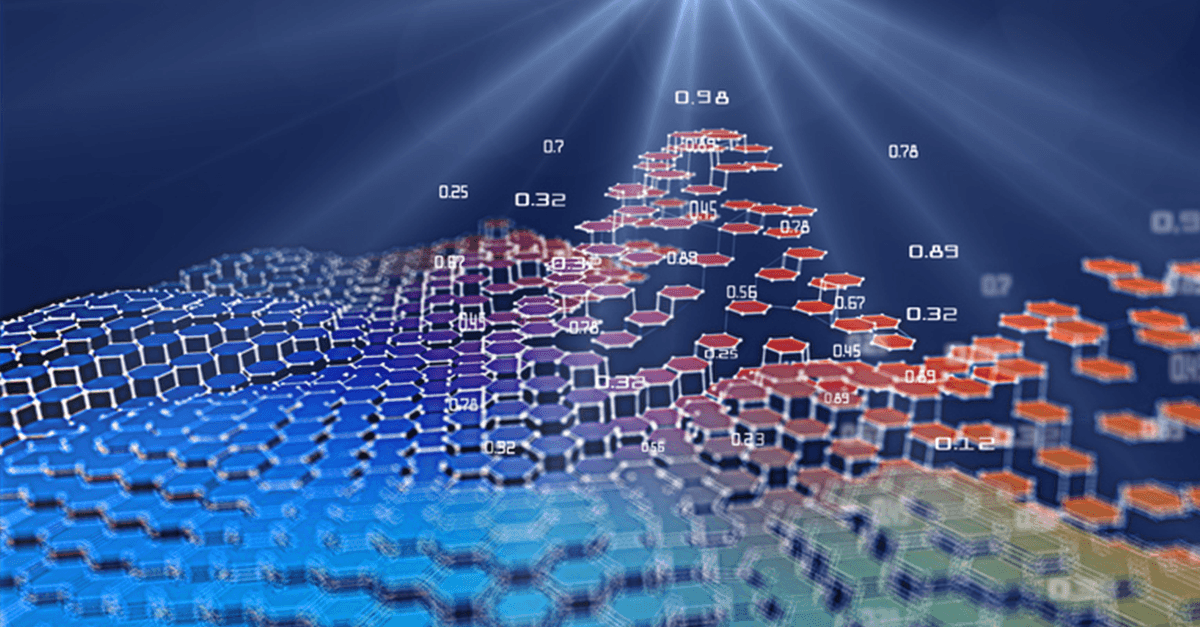
In a recent webinar with Datavant titled How AI/ML Enhance RWD For Precision Medicine, Prognos Health co-founder and Chief Medical Information Officer, Jason Bhan, MD and Chief Data Scientist, Adam Petranovich outlined how artificial intelligence (AI) and machine learning (ML) technologies are being used to add value to patient-level real-world data (RWD).
The duo set the stage by discussing the overall strengths of AI/ML as well as some of the remaining challenges associated with the technology. According to Petranovich, AI/ML tools are best suited for automating simple tasks at scale and in a fraction of the time it would take a human. Examples include email filters and classifications, text processing, document discovery, image processing, anomaly detection, and pattern recognition.
While these strengths are well documented, some key challenges including legislation limiting data rights and access, improper expectations surrounding the technology, and a shortage of data scientists continue to hinder the pace at which these tools are successfully leveraged. That being said, Petranovich firmly believes we are in a better position to capitalize on the capabilities of AI and ML today than ever before — thanks in large part to the increased availability of data, the commoditization of computing power and storage, and the consistent deployment of data modeling and frameworks.
Strengths of AI/ML in RWD
When looked at under the RWD lens, the strengths of AI/ML become more concrete. For example, the automation capabilities of AI/ML are ideal for the preparation, enrichment, and standardization of RWD. The technology can help standardize units of measure for lab tests, automatically extract ICD, CPT and NDC codes, streamline LOINC tagging, and interpret free text fields in pathology and molecular diagnostic lab tests.
From an anomaly detection perspective, AI/ML can be leveraged to flag potential clinical errors or data entry errors in patient-level RWD and detect and correct machine and lab biases. The technology can also be used to prioritize the datasets or features that add the most value to a model or process and expand patient cohorts for different clinical or commercial use cases.
How Prognos uses AI/ML
Prognos Health leverages AI and ML in a variety of ways to ensure the health data on its platform provides more value to customers.
NLP for clinical data — Prognos uses natural language processing (NLP) to abstract information and hierarchies from large clinical texts. This technology is heavily tested on lab data to detect organs, conditions, procedures, anatomical sites, and more. It is also used to automatically link clinical concepts to diagnostic codes and determine whether a patient tested positive, negative, or inconclusive for a condition (e.g. melanoma) or molecular entity (e.g. PD-L1).
Clinical data enrichment — Prognos applies machine learning algorithms to its lab data to fill in missing provider information (such as NPI), identify test value outliers for validation, and tag lab tests for hundreds of millions of records. This process helps ensure data accuracy and eliminates the need for clients to track down or verify this information themselves.
Patient utilization prediction — Prognos has also created a machine-learning algorithm that applies to its repository of laboratory, prescription, and claims information that predicts the future 12-month medical costs for a group of prospective health insurance members. This tool can help health plans quickly generate underwriting risk scores and quotes that are significantly more accurate than the industry standard.
Advanced patient insights — Using AI/ML, Prognos can predict and prioritize — with confidence — de-identified patients that will start or change therapies. This insight allows life science customers to use resources more efficiently, differentiate tactics, and prioritize PCPs to target with messaging. It also can help life science organizations identify and reach patients sooner, improving quality of life and reducing the likelihood of adverse events that could lead to hospitalization or death.
See first hand how these AI/ML investments enrich patient-level health data, leading to faster actionable insights for your life science or payer organization. Request a customized demo with one of our clinical experts or data scientists today.